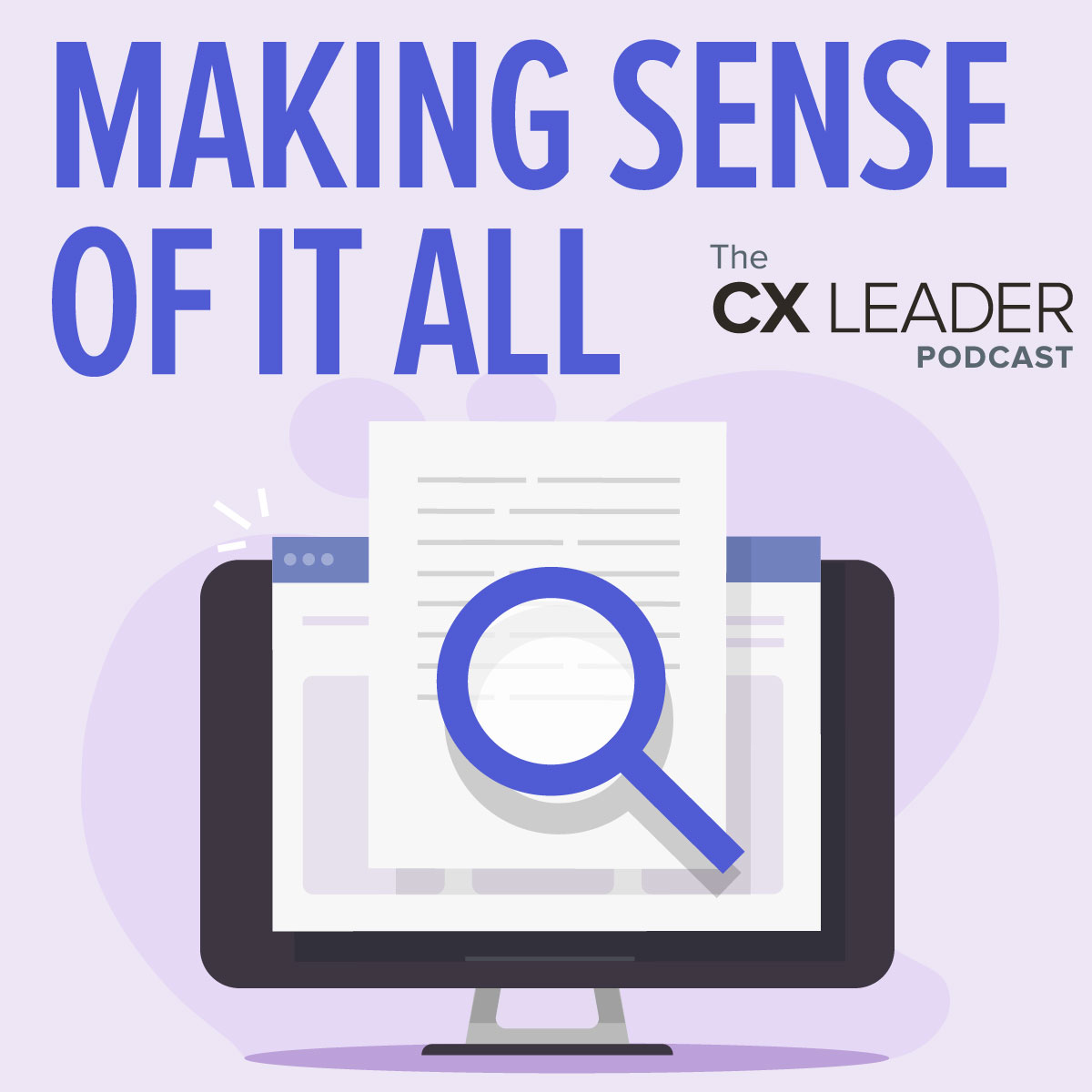
Making Sense of It All
Release Date:
Between website feedback, social media posts, product reviews, and every other way customers can provide insights, a staggering amount of data is generated everyday. If you’re a customer experience professional trying to make sense of everything your customers are saying about your company, you need a tool to help quantify all that information. That’s where text analytics can help. Host Steve Walker welcomes Matt Braun and Job Willman, both associates at Walker, to provide helpful guidance on using text analytics to quantify your data.
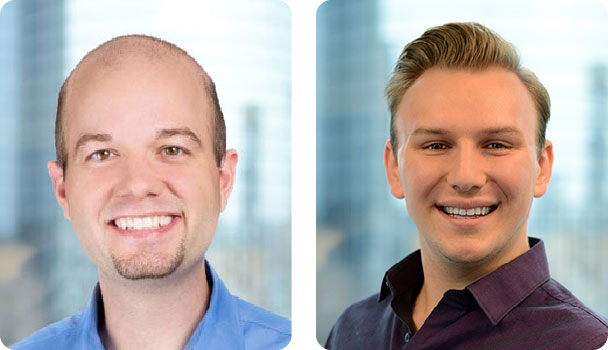
Matt Braun & Job Willman
Walker
Connect with Matt
Connect with Job
Highlights
“I do not think it means what you think it means.”
Job: “…what people should really consider is tone and expression. You know, even within maybe a short sentence, people are still conveying who they are within it and they’re all going to have their own voice. And what they say is always going it may mean different things. And I so I have a pop culture reference. I have to actually. So whenever I don’t know if anybody out there is a fan of The Princess Bride, but when the character says “inconceivable” and then Mandy Patinkin chimes in and says, “I do not think that word means what you think it means.” And sometimes when I’m looking at the data, I don’t know all of the ins and outs of a certain client. And so I need to really research what does this actually mean, because sometimes regular words can have dual meaning and they can be referencing a specific product.”
Perfectionists Beware
Matt: “Perfectionist beware when it comes to this aspect of analysis. As someone who when I first started in text analytics, you strive for one hundred percent coverage of all of your comments. You strive for perfect accuracy. And in that law of diminishing returns, that Job talked about that is absolutely it. You get to a point where where you could spend hours upon hours and a ton of resources trying to perfect this. But it is there’s very much an art and a science to what we do in text analytics. And so being comfortable with the fact that you’ve got the majority of your comments covered, that it’s accurate enough.”
Transcript
The CX Leader Podcast: "Making Sense of It All": Audio automatically transcribed by Sonix
Download the “The CX Leader Podcast: "Making Sense of It All" audio file directly.
The CX Leader Podcast: "Making Sense of It All": this wav audio file was automatically transcribed by Sonix with the best speech-to-text algorithms. This transcript may contain errors.
Steve:
It might not sound like an exciting subject, but it's a critical tool for CX professionals.
Matt:
Online reviews, social media listening, website feedback, app feedback, all of that is unstructured, qualitative information that needs to be quantified in order to make those insights really meaningful and actionable for business leaders. And so that's where we deploy text analytics to really bring that to life.
Steve:
The awesome power of text analytics on this episode of The CX Leader Podcast.
Announcer:
The CX Leader Podcast with Steve Walker is produced by Walker, an experience management firm that helps our clients accelerate their XM success. You can find out more at Walkerinfo.com.
Steve:
Hello, everyone. I'm Steve Walker, host of The CX Leader Podcast and thank you for listening. On The CX Leader Podcast we explore the topics and themes to help leaders like you leverage all the benefits of your customer experience and help your customers and prospects want to do more business with you. There's a staggering amount of data generated every day, with some estimates saying that in 2020, the average person generated approximately 1.7 seven megabytes of data every second, and over 500,000 tweets were sent each day. That's a lot of data. And if you're a CX pro trying to gain insights about what customers are saying about your company, you need a tool to help quantify all that information. That's where text analytics can help. And to discuss that, I'm happy to have on the show two of my colleagues at Walker who will help us better understand how text analytics work. Matt Braun is a vice president of advisory services. Job Willman is a project manager. It's my pleasure to have you guys on the show. This is both of your first times on the show, right?
Job:
Yeah, it is.
Matt:
Thanks for having us, Steve.
Job:
Yeah, thank you.
Steve:
You know, we're talk a little bit off the air. I have a little bit of a bias that I think the Walker people are some of the smartest CX pros out there. And we try not to overemphasize that. But I'm always delighted when I get to have Walker professionals on the podcast, because you guys really are pros. And, you know, you have so much insight with the great companies that you work with and all of our colleagues that you get to collaborate with. But maybe just for a little bit of background, if we could just maybe get both of you to tell a little bit about your own experience and your journey to the CX world. And Matt, why don't we kick it off with you and then Job you can introduce yourself just for context to our listeners.
Matt:
Yeah, thanks, Steve. And it's a pleasure to be with you today. Yeah, I've really grown up in the market research world and now evolved into a CX professional. I've been in the industry for almost 15 years, started off as a project manager myself, learning the ins and outs of research methodology and data analysis, moved into account manager roles and have really now evolved my skill set into a more rounded, CX professional focused on strategy and governance in addition to all the work that I grew up doing.
Steve:
And Job?
Job:
So I actually, prior to it working here at Walker, I didn't have any CX, you know, knowledge or experience. But starting at Walker, I really, you know, have grown up here the past almost three years now. And something, you know, that we're talking about today, text analytics has been something I've always enjoyed from the beginning. So I'm excited to talk about it.
Steve:
Well, good. Why don't we go right back to you then Job and just again, for our listeners, assuming that maybe not everybody is as knowledgeable, what do we mean when we talk about text analytics? Give us kind of the 101 their Job.
Job:
So text analytics at the most basic level is the process of categorizing open-ended feedback, where we try to take all of the comments that are made by our respondents and our customers and try to pull out thematic elements or themes from it.
Steve:
That was very nicely defined. I think you've probably done that before, once or twice.
Job:
[laughing] Maybe.
Steve:
And then, you know, Matt, on a kind of an advisory level, why would people want to employ this technique and why is this important?
Matt:
Yeah, I think is is CX professional, Steve, so much of the data that we're used to dealing with is that structured quantitative data: numbers, metrics, scales. And what we're seeing as CX evolves is that so much more of that listening is taking place outside of a traditional survey. You mentioned it in the introduction: call center notes, sales rep reports, online reviews, social media listening, website feedback, app feedback. All of that is unstructured, qualitative information that needs to be quantified in order to make those insights really meaningful and actionable for business leaders. And so that's where we deploy text analytics to really bring that to life.
Steve:
Yeah, you know, I remember some conversations… This probably goes back, you know, about the time you started your career, Matt. We worked a lot with the high tech folks in Silicon Valley and built some really sophisticated customer listening systems for some of the biggest companies. You know, right after the Internet became applicable for collecting customer data, which today sort of says, you know, "duh" but at the time we didn't know any better, so. But the tools have really evolved. You know, now to the point where I think that going back again to your comment about surveys, you know, the text analytics could actually be a replacement for some of the surveys that we've historically done like transaction surveys, right?
Matt:
Yeah, absolutely, yeah, you go back 10 or 15 years, when I started in what we call now text analytics, we're thinking about comments that are in an Excel spreadsheet and we're assigning two and three digit codes down, hundreds and thousands of comments looking at bigger topics and more granular topics, looking at positive, negative, neutral sentiment. We did all this hate say it by hand.
Steve:
Yeah.
Matt:
The platforms we have now today get you out of the gate quick in terms of topic assignments and assessing sentiment and putting it into really fancy dashboards and visualizations, we've come a long way in the last 10 to 15 years.
Steve:
So, Job, let's just say that I'm a CX pro and I haven't really leveraged text analytics or unstructured data. How would a company get started? What would be your guidance in terms of what would be the first steps you take?
Job:
Yeah. So once you've already created that listening that space to listen to your customers, the first step would be just to pull that unstructured data into some sort of database or Excel spreadsheet or, you know, some other program and then just get ingrained with it, read it, become one with the data and really try to understand what they're saying. But yeah, so first, that would really be just trying to bring that those comments, that unstructured data into one place that you can look at it in aggregate.
Steve:
And we don't make any bones about it. We're partners with Qualtrics. But what tool do we tend to use from a text analytics standpoint?
Job:
Yeah, so we use TextIQ within Qualtrics and one of the first steps you can do is from your survey, bring that into TextIQ, either within the survey platform or the dashboard platform, which I'm partial to, and then start to look at that data.
Steve:
Yeah. So you could scrape some stuff off of social media. Right. Dump it into some sort of universal file, excel or whatever you use, and then you could upload that into text IQ, right?
Job:
Yeah.
Steve:
Layman's terms, I wouldn't know how to do it, but I assume you guys do.
Job:
Yeah, there's a variety of ways you can do the Excel. You can get fancy with an API connector or just to some way get that into Qualtrics.
Steve:
And then so again, to describe sort of the state of the art and what kind of displays do you get and kind of what the power of that is, Job.
Job:
Yeah. So once you have that data and once you're looking at it, you can know within TextIQ you can search for like terms and it'll pull up a query. You can create a query string where you can search for multiple terms and that's how you really begin to build your themes. And, you know, you start to categorize the data. And once you have, like, those comments and the themes, you can refresh your data and then you can look at all of the rest of the comments that aren't categorized. And then it becomes a lot easier. And then you can go through several iterations. But that's when you really start to bring out the themes. And then once you finish that up, you can then start to visualize that within a dashboard.
Steve:
Yeah. And Matt, again, back to sort of the analytical side of this, then, can you actually start to derive some quantitative type conclusions or hypotheses from these data?
Matt:
Yeah, I think this is where it starts to get fun. You can take a look at this this text analysis at a moment in time, or you can also look at how things are trending over time. So when we talk about text analytics, I think one of the more common practices is that we're looking at what are the top three or four or five things that customers are talking about. Look at those those big topics and how they've been changing over time. You can look at not only how the volume of those comments are changing, but also how the sentiment is changing over time. That can be indicative of the kind of driver analysis that we would typically do from a quantitative standpoint. But I think there are some other things, some other use cases that aren't necessarily considered when we get started in text analytics. And that is look at the other end of the spectrum, look for some of those less frequently mentioned topics that might be growing over time that might indicate that there's an emerging issue or opportunity that you can take advantage of as a company. And then the third piece that I would mention is I think sometimes text analytics helps us rework those those sometimes static surveys that don't involve much over time. When we find that that the comments coming back or may be too broad or too specific, it can point us to where we need to make some updates in the actual survey design or listening design.
Steve:
Yeah. I'm glad you brought up the point of kind of looking for those anomalies in your data or things that maybe you hadn't considered, but starting to pop up, this is kind of totally off the subject. But I'm I'm kind of prone to do that on the show. But I was just reading earlier today, again, big demographic shift that happened in COVID is how many people acquired pets. And now the demand for veterinary services way outstrips the supply and people can't even get their pets in to see a vet. You know, again, that's something is you know, I'm just using it as an analogy, because if you can get those early on data, somebody could have predicted this, right? You know, it could have said this is going to be the downstream impact of this big change going on. And that's really part of our job as CX pros, right. Is to sort of anticipate or to say what if? And I think text analytics is just a is a great tool to kind of pop that out, right?
Matt:
Yeah, I think it helps businesses transform from being reactive to a bit more proactive.
Steve:
Yeah. And, you know, in the early days of CX, we used to talk about break fix a lot. You know, a lot of it was you were listening for what was wrong so you could go fix it. And then, you know, over time we started to say, hey, there's some opportunities on the upside. And that's exactly I think what you're describing here is how can we be ahead of things and how can we be anticipating?
Matt:
Yeah, you're looking for those those needles in a haystack sometimes, right? You're looking for those concepts, those ideas from your customers that might get legs and really help to build some momentum for your company.
Steve:
Yeah, that's great. Are there certain times Job where where you don't want to use text analytics or is there a time where you think it doesn't apply or some scenarios that it doesn't apply to?
Job:
Well, being partial to text analytics, I say always use text analytics, but there are definitely challenges you can run into when you're using text analytics. You know, like whenever you may have a large data set or you may have a really small data set. Sometimes the data set can be too small. And, you know, if you have like you can have like 20 comments, you don't really need to create themes around that. You can just read the comments. But then, you know, once you get into the upper echelon of, like, you know, hundreds of thousands of comments, it can be difficult to pull theme and meaning out from those. So I think the time is when you wouldn't use text analytics is when you have such a small data set that you can read all the comments you have maybe such a large data set and you can't actually put the time investment into it to actually make it applicable for your business.
Steve:
Hey, my guests on the podcast this week are Matt Brown and Job Willman, who are both CX pros here at Walker. And we're having a fascinating discussion about how CX pros can leverage the benefits of text analytics. Matt, from your standpoint, again, actually, it's funny that all three of us sort of have the same career path. We're just like three generations apart here. But I start as a project director like 40 years ago. But it's in some ways, a lot of this is still sort of as researchers, how we leverage quantitative and qualitative data. Right. And, you know, what's the right time to use either one in so that maybe you could just kind of for context, explain sort of from your perspective when the right times to use text analytics and when are the times maybe you don't or how do they work with the survey data, etc.?
Matt:
Yeah, yeah. So I think first and foremost, what Job is said is, has said is spot on that when you look at volume you've got to you've got to be able to weigh the time and effort that it's going to take to stand up a really complex text analytics platform with the models that that he has described so far. And when you're analyzing dozens or hundreds of comments, you know, the time and effort is greatly outweighs the potential value there. But I think the other piece that you want to be paying attention to and when it's going to be appropriate is is really twofold. One is when you're trying to probe into those why questions the w.h.y. questions. We have all of these numbers in these metrics on the dashboard, but we don't necessarily know what they mean. Well, the text analytics is going to bring that to life. And I think the second piece is humanizing the customer and the experience that they're going through. And too many times we get into dashboard reviews and offline reporting reviews and we fixate on those numbers and those metrics. And we forget that those numbers and those metrics represent our customers and either the experiences that they're going through, good, bad or ugly and in text analytics can really help to bring that to life.
Steve:
That's really a great, great description. You know, again, it's like. This cycle of quantitative and qualitative, you know, I was just as you were talking, I was thinking, you know, you know, you wouldn't have to use text analytics and in if you're just out doing like 12 or 15 executive interviews, you know, but then, you know, you could be mining your text analytics and you could find things that maybe you hadn't thought of before that then could inform maybe your next IDI's or or designed kind of some sort of survey where you could quantify that. So it's just a whole new powerful tool. OK, so what are some of the things that people need to watch out for when they're using this? What are the tricks and traps along the way job?
Job:
Yeah, so like I mentioned, the, you know, the coding and like doing any sort of, you know, trying to get to this like number, this specific number of like a percentage of the comments that you want coded. You really want to make sure that you're not putting too much effort into it. There is a law of diminishing returns where after you get to a certain percentage of your comments coded, you know, it's kind of like the effort that you're going to put into it isn't going to be the best output. So just make sure you're not putting too much effort into coding those topics.
Steve:
Yeah, and I imagine that it's you know, again, it is unstructured data. It's you know, it's not supposed to be projectable really or but it's really more for informing hypotheses and stuff. Would that be a fair statement?
Matt:
Yeah, I'll kind of sum up what Job was saying. Perfectionist beware. When it comes to this aspect of analysis. As someone who when I first started in text analytics, you strive for one hundred percent coverage of all of your comments. You strive for perfect accuracy. And in that law of diminishing returns, that Job talked about that is absolutely it. You get to a point where where you could spend hours upon hours and a ton of resources trying to perfect this. But it is there's very much an art and a science to what we do in text analytics. And so being comfortable with the fact that you've got the majority of your comments covered, that it's accurate enough. There are always going to be inconsistencies in subjectivities in this work that you can't control for. So perfectionists, beware.
Steve:
What are the things that companies ought to consider? I'll go to you first on this, Job, but what are the things that people ought to consider either before or when they're using text analytics?
Job:
Yeah, so we kind of alluded to this before about how your comments can really bring your customers to life. And I think within that, you can kind of look at the comments and strip the people away from the comments. You can just you can isolate it. And I think what people should really consider is tone and expression. You know, even within maybe a short sentence, people are still conveying who they are within it and they're all going to have their own voice. And what they say is always going it may mean different things. And I so I have a pop culture reference. I have to actually. So whenever I don't know if anybody out there is a fan of The Princess Bride, but when the character says "inconceivable" and then Mandy Patinkin chimes in and says, "I do not think that word means what you think it means." And sometimes when I'm looking at the data, I, I don't know all of the ins and outs of a certain client. And so I need to really research what does this actually mean, because sometimes regular words can have dual meaning and they can be referencing a specific product. I do have one more pop culture reference. I can sneak it in is The Princess Diaries. I know that might not be some people's area of expertise, but there is a moment whenever Julie Andrews tells Anne Hathaway that she's a princess, Anne Hathaway says, "shut up." And so Julie Andrews thinks, oh, she's telling me to shut up. But that's not right, because in the early 2000s, colloquially, we know it's like, "oh my gosh", "golly whiz", you know, that's what that means. I think you should really consider people as individuals as much as they are a part of this whole, you know, data set and really understand their tone and expression.
Steve:
You know what? We love pop culture reference on here. And it really helps illustrate the point. But you're right, it boggles the mind. But we can actually pull that out even in text. So like your examples, you'd almost have to see the face the way they intown edited it. You know, and we do know that you can use words that mean different things. Just culturally, but it's amazing that we can actually do that in the text analytics, so.
Job:
Absolutely.
Steve:
What about…
Job:
…just…
Steve:
Oh, no, go ahead.
Job:
I was just going to say that we
Steve:
Might even get another pop culture reference here.
Job:
I don't think so. I mean, you're out there. But I was just going to say that, yeah, we have to try to decipher that while just saying these words on the page and trying to consider who they are as customers or employees. So it's it can be difficult, but definitely worthwhile.
Steve:
And Matt, do you have any comments kind of on the considerations that companies should put into place before they weighed in here?
Matt:
Yes, I think we've spent a lot of time today talking about the production of the analytics itself. But what about the actual end users? Who's going to use this information and act upon it? I think one thing that you need to be considering is that CX professional inside your organization or the different consumers of that information, whether that is an executive who's looking for that top five list of things that we need to be paying attention to as an organization to guide our strategy or whether you're someone one of the feet on the street that needs to know very tactically what are the the issues or opportunities that my customers are facing. So that may mean different visualizations, some more specific, some more general. It may mean connecting it to other metrics inside of your organization, whether that's additional X-data or O-data. But just keeping in mind the different users or consumers of this information and how your visualizations are reporting might need be a little bit different.
Steve:
Gentlemen, we have reached that part of our show where I ask every guest to give us their best tip. We call it take home value. The idea here is that the CX pro, either later on today or tomorrow or next week, could take what they've learned here on this podcast and actually make some improvements in what they're doing for their organization. So I want to go first to you this time. Matt, what's your take home value for our listener?
Matt:
Yeah, I think I think one of the the summary points for today is this accordion effect of someone who is starting something basic, something small and scaling it to something large. But I would say my take home value is for someone who is at scale. Don't forget where you started, which is just reviewing those individual comments and bringing that customer to life. So my take home value would be, as you go into internal meetings, highlight a customer comment in each of those meetings, every time you get together with your team, you'd be amazed at the kinds of amazing stories that our customers tell about how we help them in a pinch or after a tragedy, how we've made their lives easier or more difficult. And I think the more that we can bring that true voice of the customer to our teams in one off individual comments, the better off will be in really understanding their journey with us.
Steve:
Thank you. That's awesome. I think that's a great way to think about how to use this inside your company to have an impact. Job, what's your take home value? What's your best tip besides movie reviews?
Job:
I would say the best is absolutely. Do spend some time with your data, but if we want to give people some guidelines about how much time and effort you should spend, if you've got a good volume of comments that, you know you want to categorize, we would say as a general rule, 70 percent of comments should be categorized and the accuracy of that is going to be about 70 to 80 percent. So just to give people numbers, because I know sometimes I really do like, oh, I need that goal to hit. So a goal, the hit would be 70 percent categorize.
Steve:
Great. Hey, my guests on the podcast this week were Matt Braun, vice president of Advisory Services, and Job Willman, a project manager also in our advisory and managed services group. Both colleagues of mine here at Walker and both passionate CX pros and and quite a bit of expertise around the topic that we had today, which was text analytics. Gentlemen, thank you for being a guest on the podcast today. Appreciate your time.
Matt:
Thank you, Steve.
Job:
Yeah, thank you for having us.
Steve:
And if any of our listeners would like to continue the conversation or connect with you, could you just give us a quick contact information, LinkedIn or your Walker email?
Matt:
Yeah. Feel free to reach out to me on LinkedIn. Matt Braun, B,R,A,U,N or you can reach out to me at mbraun@walkerinfo.com.
Job:
About the same here, Job Willman on LinkedIn. And my last name, it'll be on the podcast I assume, but the words "Will" and "man" put together. And then my email is jwillmanat@walkerinfo.com.
Steve:
Great content and very well delivered. Always proud of my colleagues and in the knowledge and the expertize that they bring each and every day. And if you want to talk about anything else you heard on this podcast or about how Walker can help your business's customer experience, feel free to email me at podcast@walkerinfo.com. Be sure to check out our website, cxleaderpodcast.com, to subscribe to the show, find all of our previous episodes. We organize them by series, topics, all sorts of interesting sorting and cohorts around our content. And you can also drop us a line, let us know how we're doing or suggest topics for a future podcast. The CX Leader Podcast is a production of Walker with an experience management firm that helps companies accelerate their XM success. You can read more about us at walkerinfo.com. Thank you for listening and we'll see you again next time.
Sonix is the world’s most advanced automated transcription, translation, and subtitling platform. Fast, accurate, and affordable.
Automatically convert your wav files to text (txt file), Microsoft Word (docx file), and SubRip Subtitle (srt file) in minutes.
Sonix has many features that you’d love including enterprise-grade admin tools, world-class support, transcribe multiple languages, upload many different filetypes, and easily transcribe your Zoom meetings. Try Sonix for free today.
Tags: qualitative data Matt Braun Job Willman text analytics tools Steve Walker